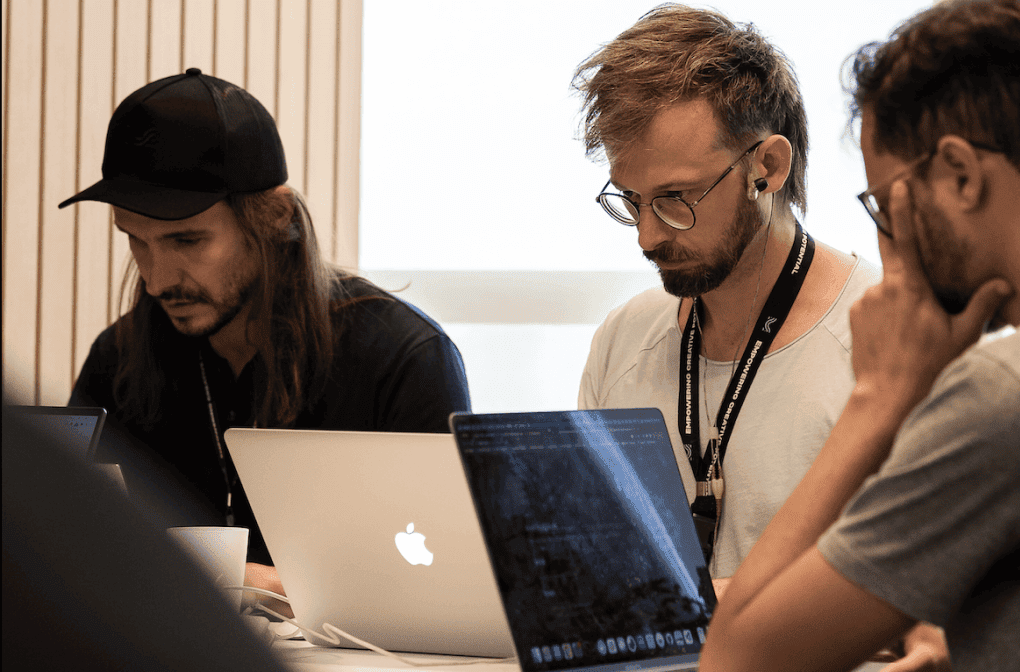
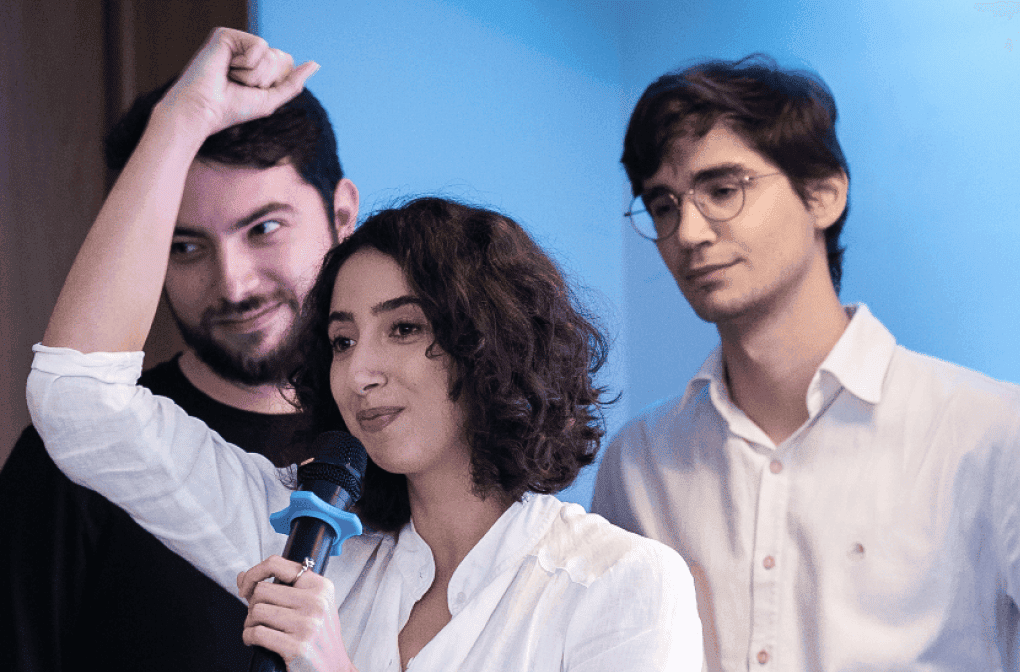
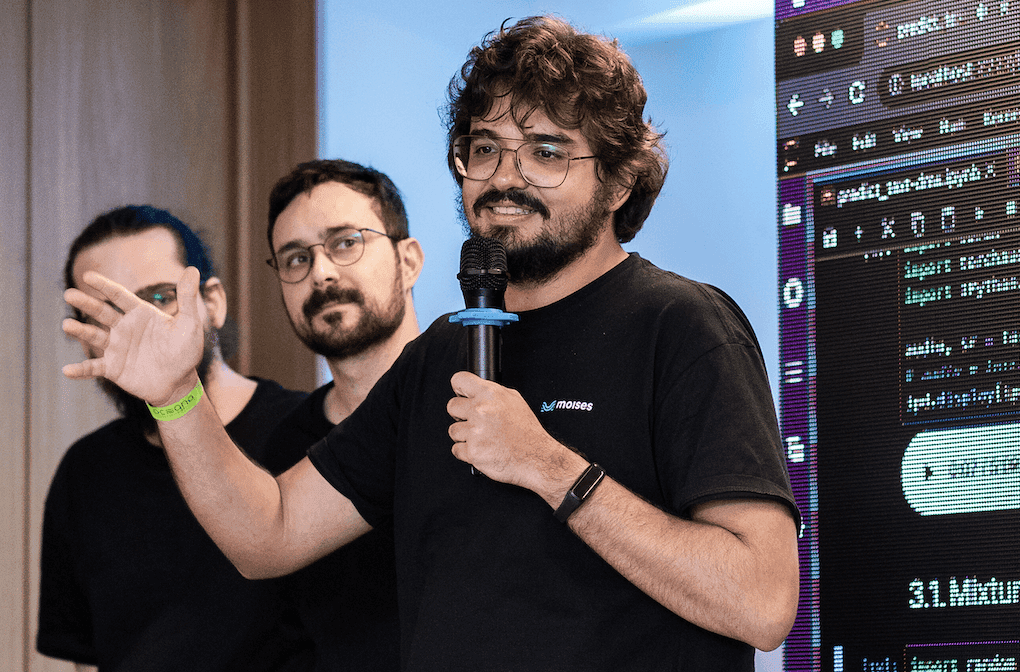
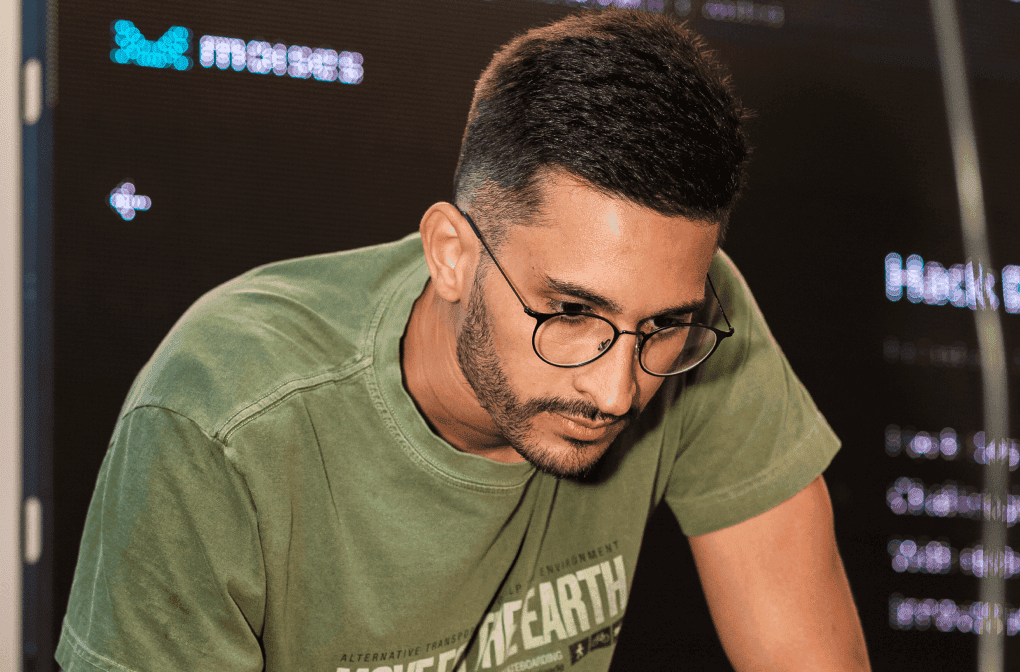
Innovation in technology knows no borders. It is a worldwide endeavor to address the intricate challenges of the music industry landscape. Our scientists and engineers embody this ethos with their diverse backgrounds and unique perspectives. We are a living proof that the collective creativity of a diverse team can compose symphonies of innovation.
The team
We're pioneering the future of music-tech with cutting-edge research. Be a part of our mission to empower creative potential through technology.
Our primary objective is to foster innovation that has the potential to empower not just individual musicians but the entire music industry as a whole.
Latest news and publications from our research team.